In this episode of Machine Learning on the Edge, host Zach Shelby is joined by EE Times senior reporter Sally Ward-Foxton, an electrical engineer with a rich background in technology journalism. Live from GTC 2024, they cover the ways that NVIDIA is impacting the AI space with its GPU innovation, and explore the latest technologies that are shaping the future of AI at the edge and beyond.
Watch or listen to the interview here or on Spotify. A full transcript can be read below.
Watch on Youtube
Listen on Spotify
Full transcript follows.
Zach Shelby
Welcome to Machine Learning on the Edge. I'm Zach Shelby, co-founder and CEO of Edge Impulse, and on this show, we're talking about data and machine learning having a huge impact on all industries. The technology is really changing the way we think about data and how we engineer solutions. We're here at GTC 2024 live. Huge pleasure to welcome Sally Ward-Foxton.
Sally Ward-Foxton
Thank you. Thanks for having me on the show.
Zach Shelby
Sally covers AI for EE Times, and you're an electrical engineer yourself. This is a huge moment for NVIDIA, for artificial intelligence, for silicon as well. And we'll get into what this all means, Jensen's big keynote in a moment. But first, I wanted to talk about you, as another electrical engineer, what brought you into the space of silicon and AI.
Sally Ward-Foxton
So it terms of career, I did my Master's in electronic engineering, University of Cambridge, so always was headed in that direction. But I've ended up where I am through several actual strokes of luck, which is usually the way. I got my first job as a journalist right out of university, and from there, basically kind of fell in love with writing about technology. Being a journalist is a really great job because it means you get to see all the brand new technologies, even before they hit the market before everybody else. You get to find out the secrets, find out what's happening first. And I really love that. It's about talking to people and finding out about people, companies and strategy as well. And I really love that angle. But yeah, I've been a journalist for about 20 years, I'm writing about electronics. That's my whole career. When I joined EE Times, five, six years ago, at first I was covering the whole of the industry, but after a while they gave me AI as a beat and it was kind of early days for AI. At first I was like "what is this? Like, what is this going to be?" I didn't realize that that one decision basically, would make my whole career.
After a while they gave me AI as a beat. I didn't realize that that one decision basically would make my whole career.
Zach Shelby
And now we have Jensen with 11,000 people at the SAP Center.
Sally Ward-Foxton
I know, the amount of attention that NVIDIA is getting and the GPUs and this technology is getting is absolutely astounding right now.
Zach Shelby
Let's talk about the Jensen keynote. My takeaway, not easily shocked, was like, wow, this is big, bigger, even bigger. And now we're transferring the entire world's internet communication capacity on a GPU backbone.
Sally Ward-Foxton
Yes, it's absolutely mind blowing. So with the new Blackwell GPU, two die right next to each other in the package, so it's twice as big as Hopper, two and a half times the performance, because they've gone to a new process load as well. So I mean, the GPU is one thing, super impressive, very, very cool. But also, when you see the racks, that's where a lot of the performance uplift is going to come from. For these generative AI models, you need to communicate across multiple GPUs, they all need to talk to each other at the same time. So Nvidia has made really big strides in the switching and the racks and so on, and the networking and communication to really boost performance. So even if even if you get two and a half x from the new GPU, we're getting more like 25 or 30x, performance uplift from the whole rack. I can't understate how much this communication bottleneck has, has been holding things back.
Zach Shelby
It almost comes to mind like this is a kind of like nation state competition to afford one of these data centers now.
Sally Ward-Foxton
I mean, it's going to be expensive. We don't know the price yet, but we know it's going to be expensive. Jensen was on stage not that long ago talking about sovereign AI and what how governments should be looking at AI and what they should be doing, using their own data with their own nuances in their culture and trying to build their own AI eyes, which I think is a really interesting point. Do you have to be a rich country to make a sovereign AI? I don't know right now. Yeah, I think we're going to start to see governments and countries building their own AI for sure.
Zach Shelby
Now as this all gets bigger and bigger, what does it mean for the edge industry? What what's the, what's the possible trickle down effect? I think of this like, space and NASA, I've always been a space person, not because not because of space in particular, I think we should take care of nature here is my own philosophy. But I find pushing the limits of technology, and then transferring that to commercial applications, what Jensen's doing is kind of similar, right? This is like pushing the limits of even physics to do Gen AI.
Sally Ward-Foxton
That's right. I mean, even like Formula One cars, that technology ends up in your own vehicle eventually. I think we're definitely going to see that effect on hardware, the kind of architecture decisions they have been making on the big scale will come some of those things that as appropriate will come to Nvidia, smaller platforms like Jetson for the robotics and so on. I think there's also, there's also an angle on when we build these really huge big AI supercomputers. That's how we do AI research today. That's how we train the biggest and the best models and state of the art models. And the more advancements we can make, and research kind of will trickle down as well to more efficient models that can run on smaller hardware and so on.
Zach Shelby
Right, so we might start to see new model architectures, like transformers, making their way more and more.
Sally Ward-Foxton
Or the next big thing, whatever that is going to be, somebody is going to invent something more efficient, we still don't know what's coming around the corner. But this research kind of drives the whole industry and you need the supercomputers to do that.
Zach Shelby
I mean, it's a great way of thinking about the the holy grail of generative AI data centers that Jensen is, is clearly driving with a vengeance, right? I'm excited to see what happens at GTC next year.
Sally Ward-Foxton
Yeah, me too.
Zach Shelby
So let's talk about generative AI as an AI workload, right, a lot of talk here about LLM and LLM is getting bigger. So the newest OpenAI, ChatGPT model, 1.8 trillion parameters. That's like exponentially growing in the complexity end. And at the same time, we have talked people talking now about small language models. Where are we going to see some of the first applications of these language models on real edge hardware, disconnected from the internet?
Sally Ward-Foxton
So I don't think we're going to see it coming to microcontrollers, necessarily, just yet. I think first places, you're going to see it on the bigger end of the edge scale. So, autonomous vehicles, maybe have some processing power, let's run an LLM so you can speak to your vehicle, access the features that your vehicle has, for example, and the car can kind of talk back to you while you're driving, I think is a really interesting use case. Anything that's a kiosk where you interact with it, and it's kind of on the edge, it's edge compute, that will get an LLM that can speak to you kind of in real language and kind of help you in real language. Anything that does concierge services or anything where you're ordering products, even restaurant ordering, those kinds of kiosks and robots will get LLMs.
Zach Shelby
We recently had a elderly care case come our way that was interested in LLMs for emergency services. What's the context of an emergency, right? Somebody's fallen down, okay, we can detect that. But what's the situation that they're in? Is there someone else there that could possibly help? How can you interact in a way that you understand what's going on for emergency services, if somebody can't speak? That was a really interesting way of using video and LLMs at the same time.
Sally Ward-Foxton
So if you have these LLMs to kind of add context to other data as well, that could be a really interesting paradigm.
Zach Shelby
Exciting. We're excited to see where generative AI goes on on the edge. Let's talk about acceleration.
Sally Ward-Foxton
Okay. My favorite topic.
Zach Shelby
Both of our favorite topics. Lot has been going on in add-on acceleration, add-on boards, M.2 boards that you're sticking into general-purpose CPUs to do more and more acceleration, I'm not quite sure for what, where that side of the industry is going. We're going bigger and bigger, are inference workloads on edge really getting that much larger? But then we have a lot of acceleration making its way into SOCs. Does this start to just become table stakes for any SOC maker that you have to have some math acceleration? Like, remember the days of cryptography, in IoT, I was involved in that.
Sally Ward-Foxton
Yes, now it's gotta be on the SoC.
Zach Shelby
There was a time where it was like, oh, no, there's one special chip that has cryptography acceleration and then it's, all of a sudden, everybody had it.
Sally Ward-Foxton
Yeah, you kind of have to have it. In terms of the M.2s that you're talking about, those are going to be around for a while, because they're going into edge boxes and appliances where it's maybe aggregating video feeds for a security camera or something. So it's a bigger installation. It's not edge endpoint kind of edge. At the endpoint, we are seeing separate smaller accelerators with these novel architectures, which is super cool and super fun for me to write about.
Zach Shelby
Will they be distributing the workload within the edge machine?
Sally Ward-Foxton
Yeah so let's have an accelerator from BrainChip or Syntiant or somebody where it's a separate chip and it's alongside your CPU or or your microcontroller. I think eventually, a lot of that will go down the same route that you're talking about for cryptography, we'll go on to the SOC. It will be a block on the SOC, because that makes the most sense for embedded use cases for power efficiency and integration and so on. We will get there eventually. Not today, but eventually.
Zach Shelby
Very interesting. We have a good example of what's happened in the industry now with this little box, this is just an AI-powered camera reference design that we helped build, with a bunch of different partner and customer cases. One of those being nature conservation. So it turns out that a lot of endangered species, poaching, human conflict, nature conservation cases like elephants in Africa, really can make use of computer vision, with AI, in the field. Deep in the forest, in the jungle, needs to be left there for months at a time, very hard to go collect data. This has no acceleration. This is an STM32H7. So high-end Cortex M7, a lot of external memory, so we can put models that range from 10 to 20 megabytes in size, even, into this. And with software techniques, quantization, compression, re-architecting some of the ways that we do object detection, we can do fairly real-time object detection on this, and because it's a microcontroller architecture, we can do that for a year of battery life, with a certain number of PIR events per day where we capture images. And that's what no acceleration. So it's really interesting, as we get acceleration into these types of existing SOCs, say the next generation of ST microcontrollers has an accelerator, where's that going to get us and bring us right? What's the kind of optimization we should be thinking about from a from device manufacturers point of view? Like, all right, I've got a camera. Yep. We don't have acceleration, we're going to do a little bit of AI. Now we're going to want to add acceleration for the next generation.
Sally Ward-Foxton
Yeah, I mean, if you're looking at reducing power, doing more efficient ML, you definitely need acceleration today, I guess it's a balance of can your application handle the extra cost that you're going to face?
Zach Shelby
We certainly could burn through the interference faster with an accelerator, that would allow us to go back to sleep faster. Improve our sleep duty cycle, because low power modes in microcontrollers can go very low. I could also see the need for always on, we talked about Syntiant a little while ago, that's really innovative in the way that we can really run always on. Say, instead of a passive infrared sensor, we could do something with like an audio detection. We heard an elephant, rather than saw random movement, a lot of false images that way, I could see that always-on capability be really interesting too.
Sally Ward-Foxton
Yeah, there are a lot of, there are several at least, of always-on type of accelerators on the market. So start picking the right one for you. A lot of those are analog, which is very exciting too. But yeah, an emerging market let's say.
Zach Shelby
I want to talk more about these applications and industries. We touched on a few things like automotive, we're seeing the most forward-looking companies right now, across medical devices, energy, manufacturing, logistics, and warehouses — we have a little warehouse application running behind us — they are adopting artificial intelligence into their edge systems and embedded products. But it's like the very early wave of adoption. What's holding us back? Why can't, what doesn't all the industries adopt this stuff much faster?
Sally Ward-Foxton
Yeah it will take time. There are a number of things, I think, that are really coming together to kind of crash everything. I mean, software, as always, the hardware is emerging, and it's working, which is great. But software, software vendors, software stacks, I think some of those, definitely, there's room for improvement. As those mature as well, things will get a little easier. Education, people are developing these kinds of products, they're not necessarily data scientists or ML engineers, and they're going to have to be, so there's a level of education required as well. There are also things for commercial deployments. I think we're not quite there yet, to help you deploy at scale. How do you do that today with something that is an emerging technology? How do you handle product lifecycle, how do you update in the field? All of these some of these are bigger question marks and others but they're still question marks right now. So yeah, as things are maturing, we're trying to sort out these problems, but I don't think we're quite quite there yet.
Zach Shelby
I agree with all that. In addition, like we're seeing a lot of problems with "how do we get hold of the data?"
Sally Ward-Foxton
Right? Yeah, where does it come from in the first place?
Zach Shelby
"What is useful data?" Right? Like what kind of data can you apply to AI versus applying it to maybe a normal engineering process that's code based? So, where does useful AI data come from? How much of it do you need? And then how do you get started, get into a deployment, and then use that deployment to collect more data? That's actually one of the more interesting paradigms I've been seeing lately, this idea of an active learning loop. Where, don't try to spend half a year or a year making the world's largest dataset of whatever, right? You know, a million samples of this or that, before you even try to deploy it in a real system, because it might not even work. That's expensive, right? Building datasets. So why don't we start small, put a small model there, and then use that model to detect when you don't do something right, right? What are the things that you miss? Let's take samples of that data, push that back into your data set, start auto-labeling and testing. Can we use that data to improve the accuracy of this model? Yeah, super interesting.
Sally Ward-Foxton
I think we're making strides on augmenting with synthetic data as well, right at the moment, which can help. But again, I think it's still developing, although you know even more about that than me.
Zach Shelby
Well, a lot of talk about Omniverse here at GTC. And we're finding that this kind of digital twin type of environment is very useful for synthetic data generation, especially in industrial environments where we're working with factories, for example, that haven't been built yet. Oil and gas facilities that are so remote you need to fly a helicopter to go collect data. That's expensive, right? So, really difficult environments, some difficult to go measure. This warehouse example behind us, that was built all with Omniverse. That's entirely Omniverse modeled, because the warehouses are actually really hard to go out to, they're far away, it's expensive to send a team to go collect data. So instead, one of our engineers was fed up of traveling to the warehouse, created an Omniverse model himself, and then we have a connector just feed that data into a data set. So that entire data set is Omniverse generated. And then we can train a model and then deploy it back to the Omniverse model. And test it, lots of different lighting conditions, angle conditions, get a more robust model. I think there's some there's a lot to this kind of like digital twin usage in synthetic data and like creating better models. super interesting.
Sally, thank you so much for being on the show.
Sally Ward-Foxton
Thank you, thanks for having me on the show.
Zach Shelby
Thank you for joining us on Machine Learning on the Edge. We welcome you to our next episode. And please join us at GTC next year.
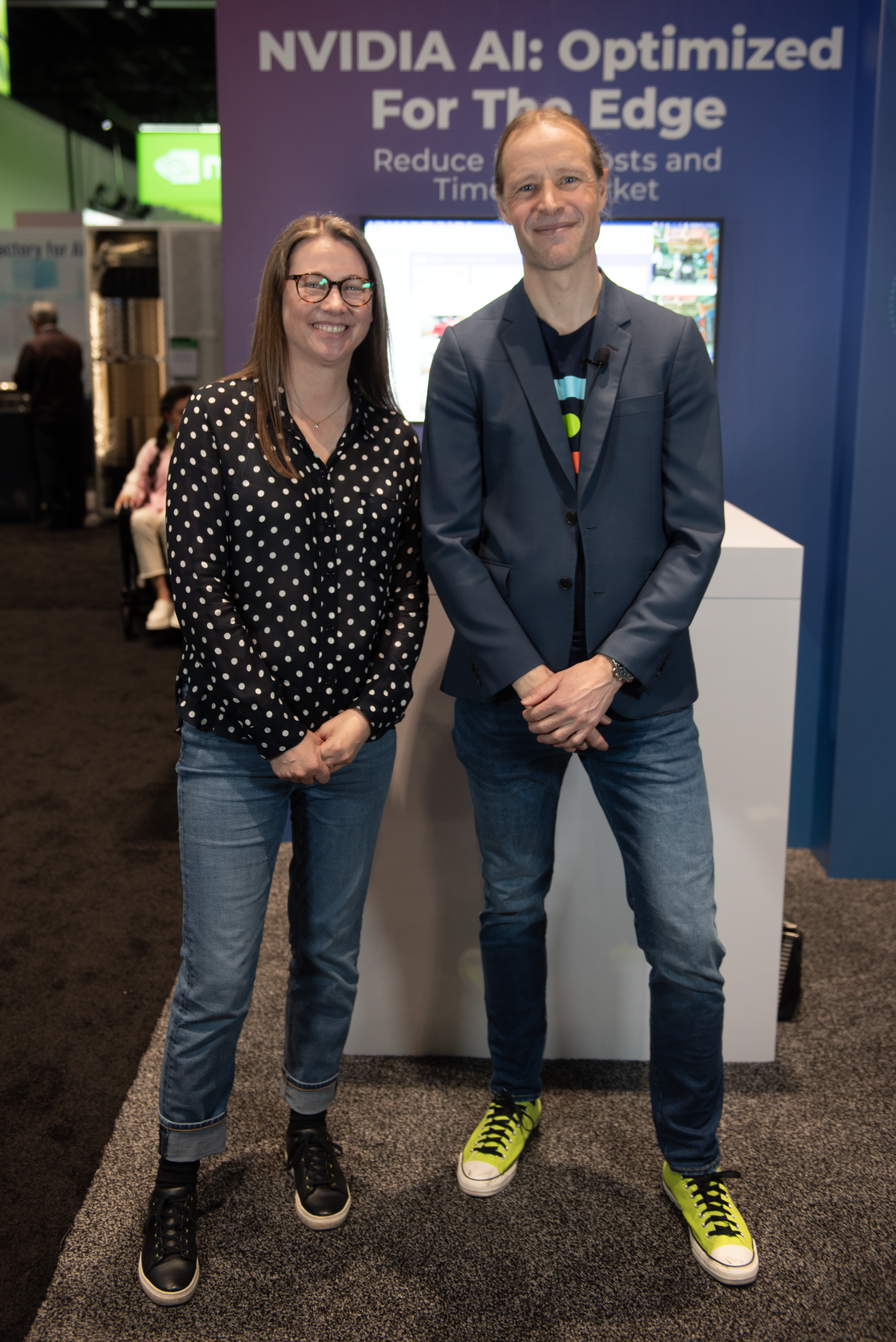